Unlocking the Potential of Big Data for Business Decisions
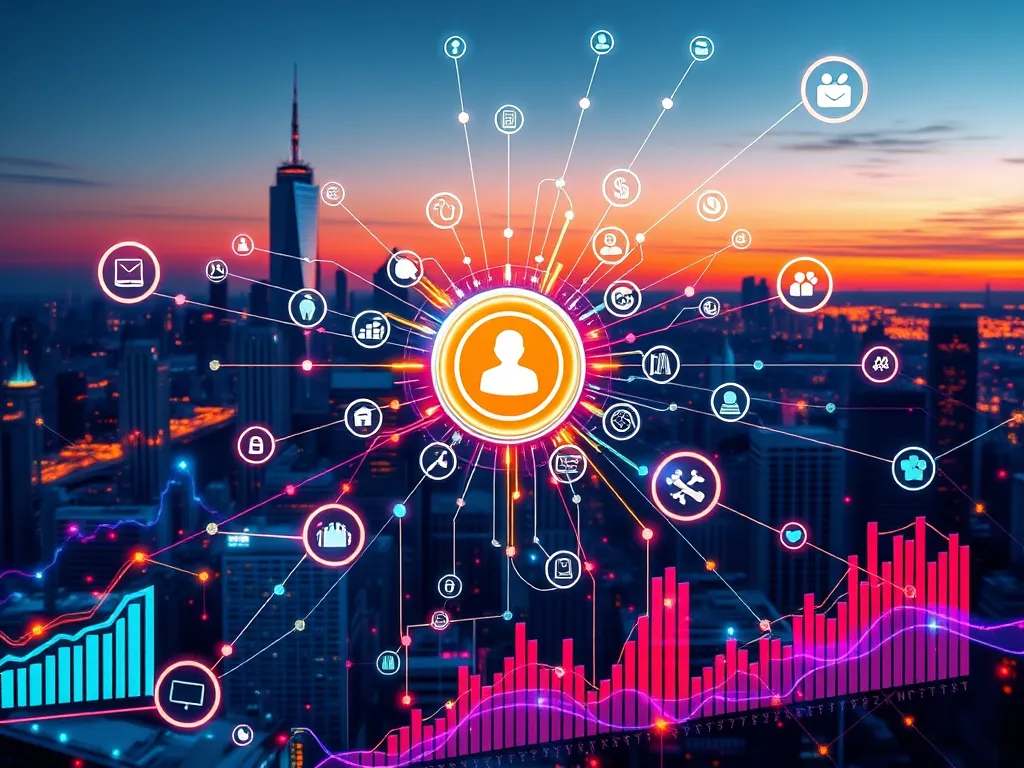
Understanding Big Data Analytics: Transforming Data into Insights
Big Data Analytics is a powerful mechanism that enables organizations to analyze vast amounts of data for meaningful insights. Utilizing advanced algorithms and statistical methods, businesses can uncover patterns, trends, and correlations in data that were previously hidden. This process is essential in a world where data is generated at an unprecedented rate, and traditional data processing methods are no longer sufficient to handle the scale and complexity of the information.
The importance of Big Data Analytics lies in its ability to provide actionable insights that can drive strategic decision-making. Organizations leverage analytics to better understand customer behaviors, optimize operational processes, and enhance product offerings. With the right analytical tools and techniques, businesses can turn raw data into a strategic asset, which can significantly improve their competitiveness in the marketplace.
In today’s digital landscape, Big Data Analytics offers opportunities for innovation and growth across various industries. From healthcare to finance, and retail to manufacturing, organizations are harnessing the power of data analytics to not only meet but exceed their business objectives. This analytical approach helps organizations remain agile and responsive in an ever-evolving marketplace, catering to the demands and preferences of their customers effectively.
Ultimately, Big Data Analytics encompasses a broad range of technologies and methodologies that empower businesses to make data-driven decisions. Organizations that adopt advanced analytical capabilities are better positioned to identify emerging trends, forecast future demands, and drive sustainable growth, ensuring they stay ahead of the competition. With continual advancements in technology, the landscape of Big Data Analytics is poised to evolve further, unlocking even greater possibilities.
This article explores the fundamental aspects of Big Data Analytics, including its definitions, techniques, applications, tools, challenges, and future trends, providing a holistic view of how organizations can capitalize on the power of data to transform their businesses.
Understanding Big Data
Big Data refers to data sets that are so voluminous and complex that traditional data processing applications are inadequate to handle them. The definition of Big Data extends beyond just the volume of data; it encompasses various characteristics known as the 'three Vs': Volume, Velocity, and Variety. Volume relates to the immense amounts of data generated, Velocity refers to the speed at which the data is generated and processed, and Variety denotes the diverse types of data, including structured, semi-structured, and unstructured formats.
Big Data can be categorized into different types, including structured data, which is organized in a predefined manner (such as databases); semi-structured data, which has some degree of organization (like XML or JSON files); and unstructured data, which lacks a specific format (such as text documents, images, or social media posts). Understanding these types is crucial for businesses as they design their data strategies to capture, store, and analyze information effectively.
The role of Big Data in modern businesses is transformative. It allows organizations to gain deeper insights into customer preferences, monitor operational efficiencies, and adapt to market changes rapidly. By leveraging Big Data, companies can create personalized marketing strategies, enhance customer experiences, mitigate risks, and drive innovation. Essentially, it enables organizations to make informed decisions based on data rather than intuition alone.
Big Data Analytics Techniques
Descriptive analytics is one of the primary techniques used in Big Data Analytics. This method analyzes historical data to expose trends and patterns over time. By collating and summarizing past data, businesses can gain insights into what has occurred, which helps them understand their past performance and make comparisons over various time periods. This type of analysis is critical as it provides a foundation for further analytical methods.
Predictive analytics takes it a step further by utilizing statistical algorithms and machine learning techniques to analyze historical data and predict future outcomes. This form of analytics can forecast customer behaviors, market trends, and other essential business metrics. Businesses can use this insight to improve decision-making and develop proactive strategies that capitalize on projected market changes.
Prescriptive analytics offers organizations strategic recommendations based on data analysis. It involves using optimization and simulation algorithms to offer solutions for the best course of action. By analyzing various scenarios and potential outcomes, prescriptive analytics helps businesses to make sound strategic decisions that improve efficiency, cost-effectiveness, and overall performance, ensuring they are well-positioned for the future.
Applications of Big Data in Business
One of the primary applications of Big Data Analytics is customer segmentation and targeting strategies. Organizations analyze customer data to create personalized experiences and identify niche markets. By segmenting their audience based on behaviors, preferences, or demographics, businesses can effectively tailor marketing efforts, increase engagement, and drive sales conversion rates.
Big Data also greatly influences product development. By analyzing customer feedback, market trends, and competitors' performance, businesses can make more informed product improvements or innovations. Moreover, analytics enable companies to identify gaps in the market and align their product offerings with customer demands, fostering a customer-centric approach to product development.
Enhancing operational efficiency is another significant application of Big Data in business. Organizations analyze operational data to identify bottlenecks, inefficiencies, and waste in their processes. This insight allows them to streamline operations, optimize resource allocation, and ultimately reduce costs. By utilizing Big Data to drive operational improvements, organizations can achieve higher productivity and profitability.
Big Data Tools and Technologies
There is a wide array of tools and technologies available for Big Data Analytics. Some popular tools include Apache Hadoop for distributed storage and processing, Apache Spark for fast data processing, and Tableau for data visualization. Additionally, platforms such as Google BigQuery and Amazon Redshift provide cloud-based solutions for managing and analyzing large data sets. Understanding the right tools is crucial for organizations in developing effective data strategies.
When it comes to data processing frameworks, organizations often choose between batch processing and stream processing. Batch processing frameworks, such as Hadoop, are customized for processing large datasets at a scheduled interval. In contrast, stream processing frameworks like Apache Kafka and Apache Flink are built for real-time data collection and analysis. A comparative analysis of these frameworks is essential for businesses to determine which approach aligns best with their data needs.
Emerging technologies in Big Data Analytics, such as Artificial Intelligence (AI) and Machine Learning (ML), are changing the landscape of how data is analyzed. These technologies enhance analytical capabilities by automating data processing and improving predictive modeling accuracy. Furthermore, advancements in natural language processing (NLP) enable organizations to analyze unstructured data efficiently, unlocking insights from a broader range of sources.
Challenges in Big Data Implementation
One of the most pressing challenges in Big Data implementation is data privacy and security. As businesses collect and analyze vast amounts of personal and sensitive data, they must ensure compliance with regulations such as GDPR and CCPA. Moreover, organizations face the challenge of protecting their data from breaches and cyber-attacks, necessitating robust security measures to safeguard their data assets.
Technical limitations and resource requirements can also hinder Big Data implementation. The necessity for advanced technology infrastructure, skilled personnel, and financial resources can be overwhelming, particularly for smaller organizations. Selecting the right tools, investing in training, and ensuring data quality can pose significant challenges that organizations must navigate to reap the benefits of Big Data Analytics.
Furthermore, organizational resistance to Big Data adoption may arise due to a lack of understanding about the capabilities and value of analytics. Employees may feel threatened by data-driven decision-making or may resist adopting new analytical tools and processes. Organizations must foster a culture of data-driven decision-making and invest in change management to overcome these barriers and promote the successful adoption of Big Data Analytics.
Future Trends in Big Data Analytics
The impact of Artificial Intelligence and Machine Learning on analytics will be profound. As these technologies advance, they will enhance analytical capabilities, improve predictive accuracy, and automate data processing tasks. This evolution will enable organizations to derive faster insights and adapt to market changes in real-time, establishing a more dynamic approach to Big Data Analytics.
Trends in real-time data processing will continue to grow as businesses increasingly require immediate insights to respond to customer needs and market dynamics. Real-time analytics will allow organizations to harness streaming data, providing them with the ability to react swiftly to changes and make timely decisions that enhance customer experiences and operational outcomes.
The growing importance of data governance and quality management will also shape the future of Big Data Analytics. As organizations gather more data, the need for effective data governance frameworks and processes will become critical in ensuring data integrity, quality, and compliance. Organizations must prioritize data governance to establish a sustainable Big Data strategy that drives long-term success.